The Neighborhood Effects project investigates the implications of neighborhood characteristics for political behavior, taking particular care to distinguish between objective conditions of the neighborhood (e.g. percent in poverty) and subjective conditions of the neighborhood (e.g., presence of graffiti). In addition to creating exceptionally rich data (the PhillyStreets dataset), this project introduces a convolutional neural network model to predict demographic information (e.g., race and income) from neighborhood images and illustrates systematically the physical characteristics that are important for predicting demographic makeup.
We realized that undergraduates are eager to be involved in research but may not know how to get involved in a project. A significant motivation for creating ML4PEAL was to connect faculty and these students around research projects, fostering awareness of the kinds of research in which social science and computer science faculty are engaged in together. We thank the Council for Science and Technology for their support.
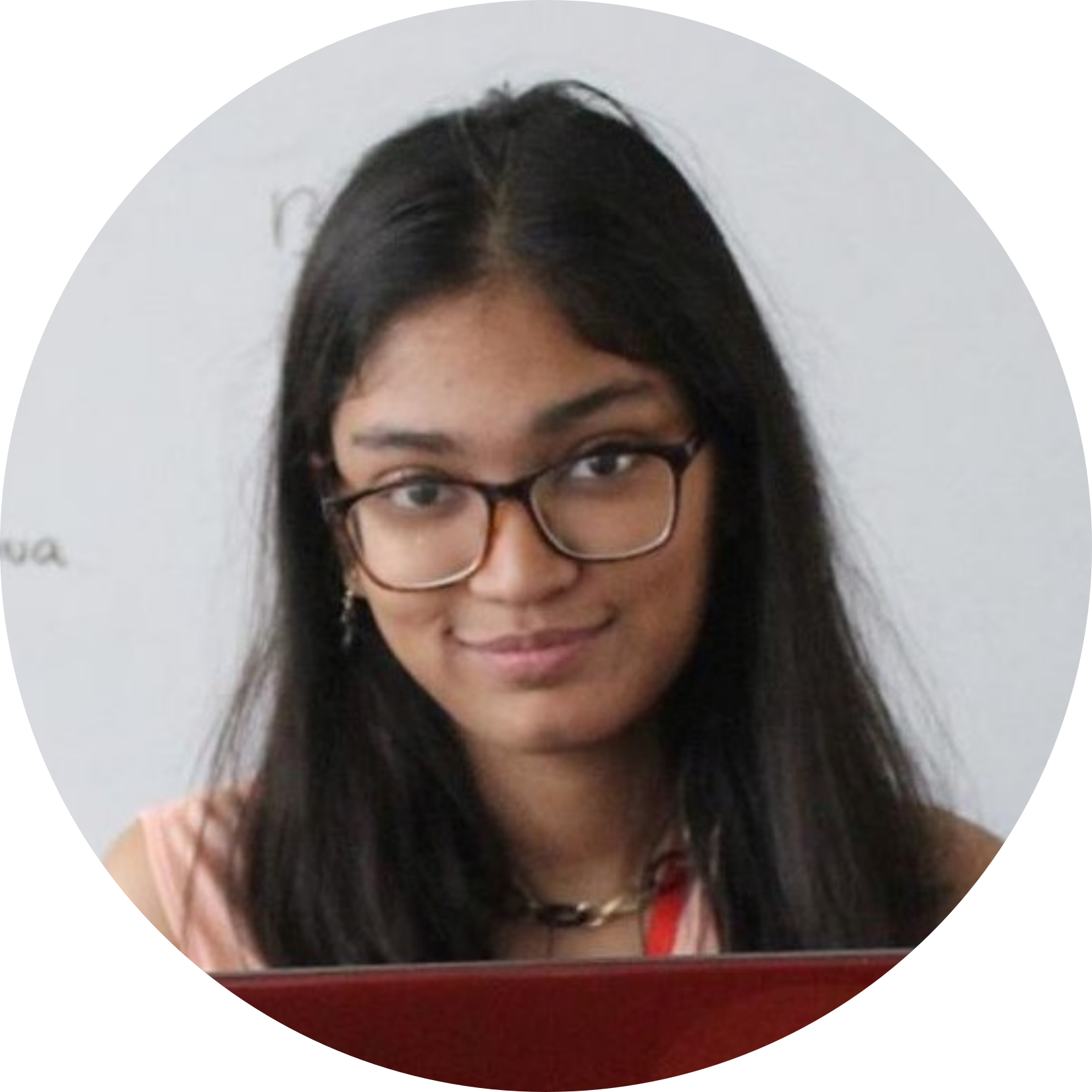
Mapping and Analysis RA
"[I'm] excited to use maps and data analysis to make elusive issues of racial inequality easier to see and understand in everyday life"
Imaan's work in ML4PEARL consists widely of spatial analysis on racial and income inequality across US neighbourhoods, through mapping (in GIS) and statistical analysis (in R and Excel).
An example of their work is shown here.
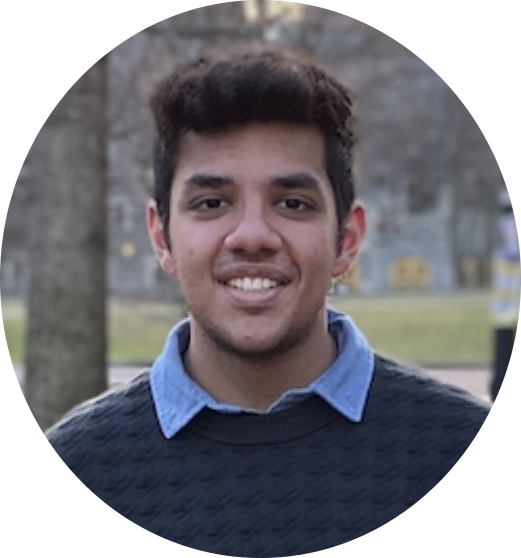
Computer Vision RA
"[I] particularly [enjoy] drawing on concepts from classical physics and control theory to explore new ideas"
Abhinav's work at ML4PEARL focuses on dataset generation (writing scripts to collect google street view images over a given city) and computer vision (training PyTorch based neural network to make predictions based on neighborhood images)
An example of their work is shown here.
The PhillyStreets dataset is a collection of over 100k Google Street View images collected over the city of Philadelphia, PA, and their demographic information (e.g., race and income makeup). This dataset was used to train a neural network model to predict objective conditions of a neighborhood (e.g., percent poverty) from neighborhood images.